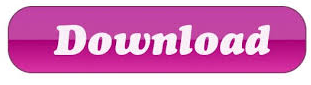

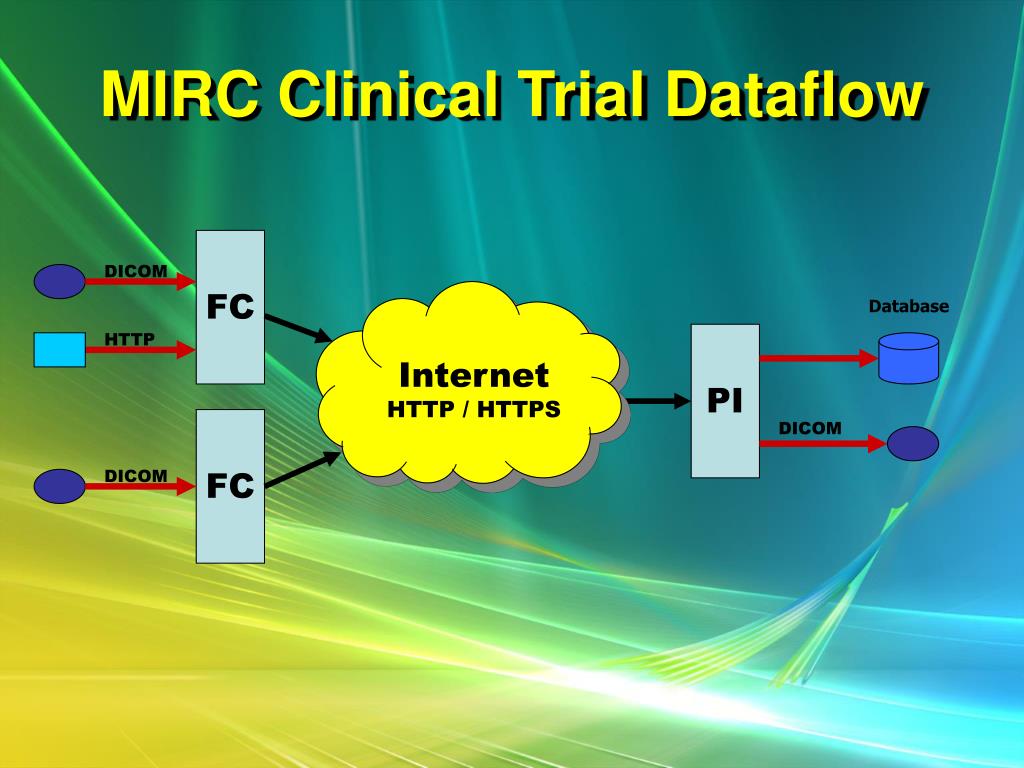
The simulation results indicate that agents can successfully learn and generate human-like travel activities. We applied the model to the data collected from a smartphone application and attempted to replicate a large amount of the population’s daily movement by incorporating with agent-based multi-modal traffic simulation technologies. Unlike traditional travel demand modeling approaches, our method focuses on the problem of inferring the spatio-temporal preferences of individuals from the observed trajectories, and is based on inverse reinforcement learning (IRL) techniques. In this study, we developed a reinforcement learning-based approach for modeling and simulation of people mass movement using the global positioning system (GPS) data. In contrast, emerging data collection methods have enabled researchers to leverage machine learning techniques with a tremendous amount of mobility data for analyzing and forecasting people’s behaviors. However, researchers have developed travel demand models to accomplish this task with survey data that are expensive and acquired at low frequencies. Understanding individual and crowd dynamics in urban environments is critical for numerous applications, such as urban planning, traffic forecasting, and location-based services.
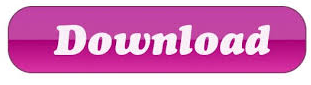